20 January 2023
Simplify – Advanced Machine Learning to support balancing the system
Keeping the balance in the grid is essential: the injection of power must always be equal to the consumption to keep the frequency at 50Hz. Due to the increasing penetration of limitedly predictable renewable energy, balancing the system could become increasingly challenging.
Why this project
To maintain or restore the balance in the system, Elia relies on two pillars. The first pillar consists in providing market parties with the correct incentives to maintain the balance in their own portfolios and/or to deviate from a balanced portfolio in order to support balancing the system. The second pillar consists of so-called balancing reserves that are contracted by Elia to resolve residual imbalances. These balancing reserves consists of a variety of assets that can increase/decrease their injections/offtakes from the grid upon request of Elia to restore the balance in the system.
To keep the costs related to balancing the system as low as possible, it is essential that accurate forecasts can be made of the system imbalance in the coming minutes/quarter hours such that both market parties and Elia can take the appropriate actions for balancing the system. However, this is not straightforward as the system imbalance is the result of complex interactions between different events. On the one hand, these could be events that are by nature very difficult to predict, such as forced outages of generation units and wind and solar generation forecasting errors. On the other hand, the system imbalance will also depend on the response from market parties to perceived or anticipated imbalances, their trading and balancing strategies as well as their available flexibility. As a result, the system imbalance can be highly volatile and difficult to predict even on short time frames (as illustrated in the graph below).
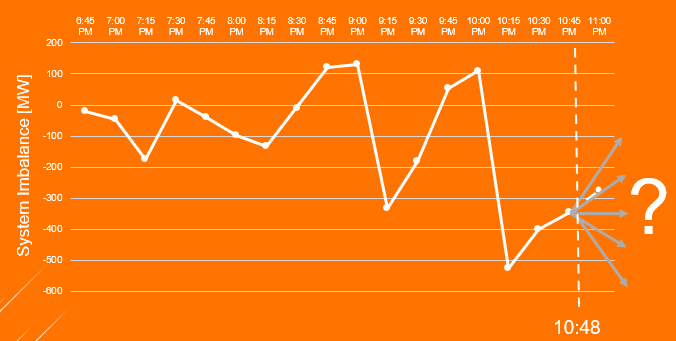
In this context, the objective of the Simplify project is to design and industrialize machine-learning model(s) to forecast the system imbalance in the coming minutes/quarter hours, and to make these forecasts available to the market parties and to Elia’s National Control Centre to support decision-making on balancing actions.
Approach
Following pioneering work started in 2019, Elia performed a detailed study in 2021 to develop, test and evaluate different machine-learning models to forecast the system imbalance. Based on multiple years of historical data, Elia experimented with the input data (predictors) as well as the type of machine-learning models that would provide the best possible forecasts. Towards the end of this study, a proof-of-concept was developed to test the developed model in an operational, near real-time environment.
Following the results of this study, it was decided to industrialize the developed tool to forecast the system imbalance. In parallel, Elia has continued its efforts in 2022 to look for further opportunities to further improve the accuracy of the system imbalance forecasts and will remain to explore further opportunities in 2023.
Results
The performance of the system imbalance forecasts is mainly evaluated based on the root mean square error (RMSE), which shows to what extent the forecasted system imbalance deviates from the actual system imbalance.
Following repeated model improvements, the RMSE of the SI forecasts for the next quarter hour have been reduced from an initial 170 MW to around 100 MW.
However, Elia is aware of the fact that there is still a significant error margin around the provided forecast. For this reason, Elia does not only provide a point forecast of the system imbalance but also provides information related to the expected probability that the system imbalance will lie within certain intervals.
Since October 2022, the forecasts of the system imbalance in the ongoing quarter hour and the next quarter hour are made available in real-time on the EliaOpenData portal. Here, all stakeholders can directly access the data.
In addition, more information regarding the machine-learning models effectively deployed are made available on the Elia website.